
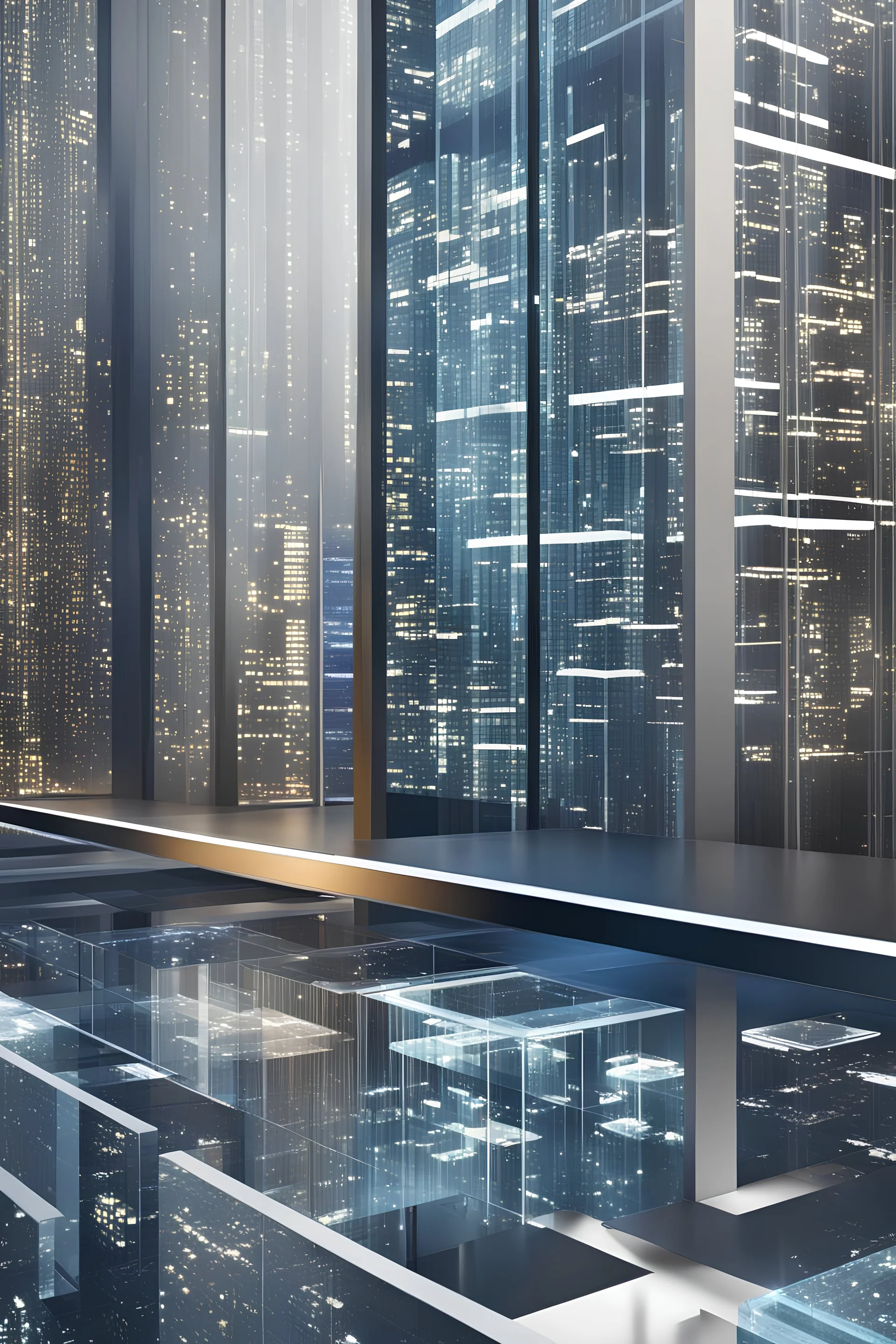
@generalpha
Prompt
Data selection and cleaning are essential in reducing parameters in a model, especially in neural networks. Selection focuses on relevant and representative samples, reducing dataset size and mitigating overfitting. Cleaning removes noise and inconsistencies, improving data quality and model performance. By selecting informative data, we achieve similar or better results with fewer parameters. Cleaning further simplifies the problem space, reducing dimensionality and improving computational effi
doubles, twins, entangled fingers, Worst Quality, ugly, ugly face, watermarks, undetailed, unrealistic, double limbs, worst hands, worst body, Disfigured, double, twin, dialog, book, multiple fingers, deformed, deformity, ugliness, poorly drawn face, extra_limb, extra limbs, bad hands, wrong hands, poorly drawn hands, messy drawing, cropped head, bad anatomy, lowres, extra digit, fewer digit, worst quality, low quality, jpeg artifacts, watermark, missing fingers, cropped, poorly drawn
1 year ago
Model
SSD-1B
Guidance Scale
7
Dimensions
3328 × 4992