
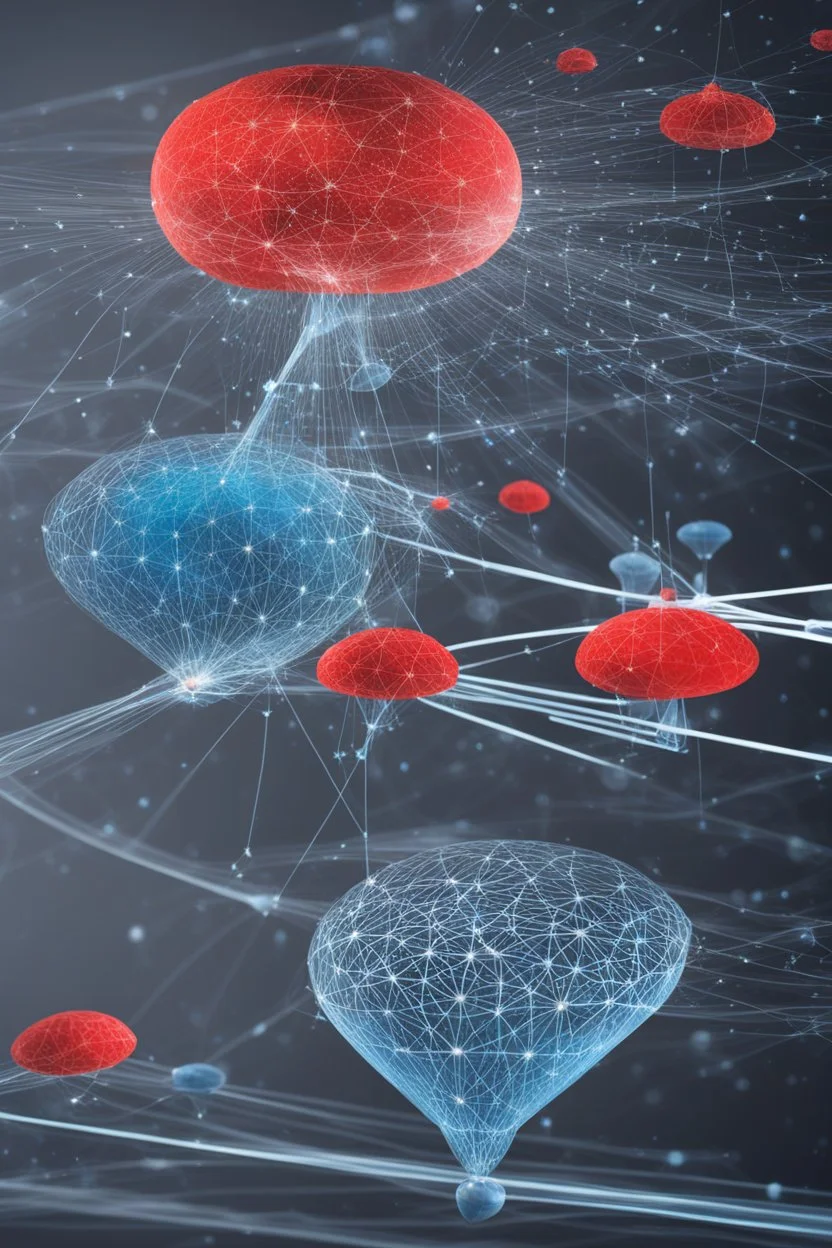
@generalpha
Prompt
Spurious correlations can occur in machine learning when the data collection process is influenced by uncontrolled confounding biases. These biases introduce unintended relationships into the data, which can hinder the accuracy and generalization of learned models. To overcome this issue, a proposed approach involves learning representations that are invariant to causal factors across multiple datasets with different biases. By focusing on the underlying causal mechanisms rather than superficial
doubles, twins, entangled fingers, Worst Quality, ugly, ugly face, watermarks, undetailed, unrealistic, double limbs, worst hands, worst body, Disfigured, double, twin, dialog, book, multiple fingers, deformed, deformity, ugliness, poorly drawn face, extra_limb, extra limbs, bad hands, wrong hands, poorly drawn hands, messy drawing, cropped head, bad anatomy, lowres, extra digit, fewer digit, worst quality, low quality, jpeg artifacts, watermark, missing fingers, cropped, poorly drawn
1 year ago
Model
SSD-1B
Guidance Scale
7
Dimensions
832 × 1248