
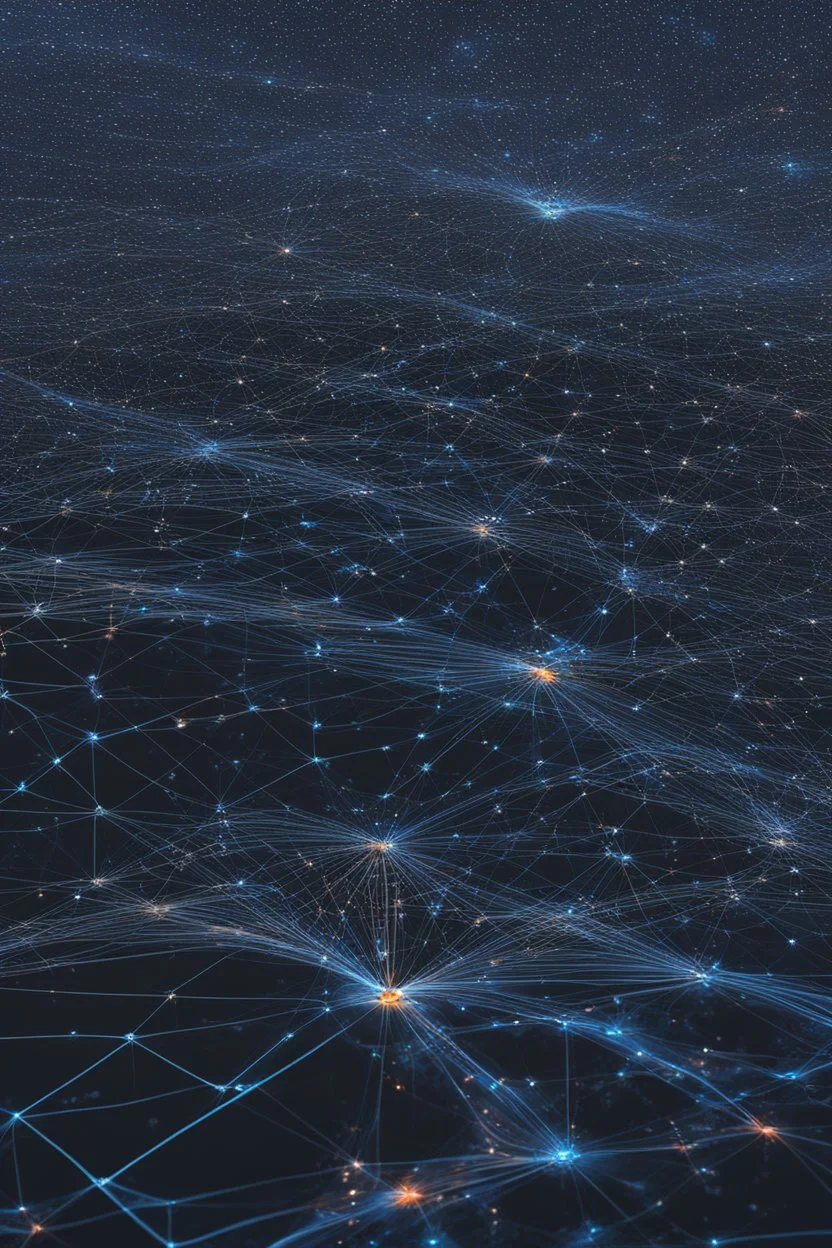
@generalpha
Prompt
Local and global approaches in mathematics and machine learning are both universal approximators, but they differ in the number of parameters required to represent a given function accurately. The entire system, including data, architecture, and loss function, must be considered, as they are interconnected. Data can be noisy or biased, architecture may demand excessive parameters, and the chosen loss function may not align with the desired goal. To address these challenges, practitioners should
doubles, twins, entangled fingers, Worst Quality, ugly, ugly face, watermarks, undetailed, unrealistic, double limbs, worst hands, worst body, Disfigured, double, twin, dialog, book, multiple fingers, deformed, deformity, ugliness, poorly drawn face, extra_limb, extra limbs, bad hands, wrong hands, poorly drawn hands, messy drawing, cropped head, bad anatomy, lowres, extra digit, fewer digit, worst quality, low quality, jpeg artifacts, watermark, missing fingers, cropped, poorly drawn
1 year ago
Model
SSD-1B
Guidance Scale
7
Dimensions
832 × 1248