
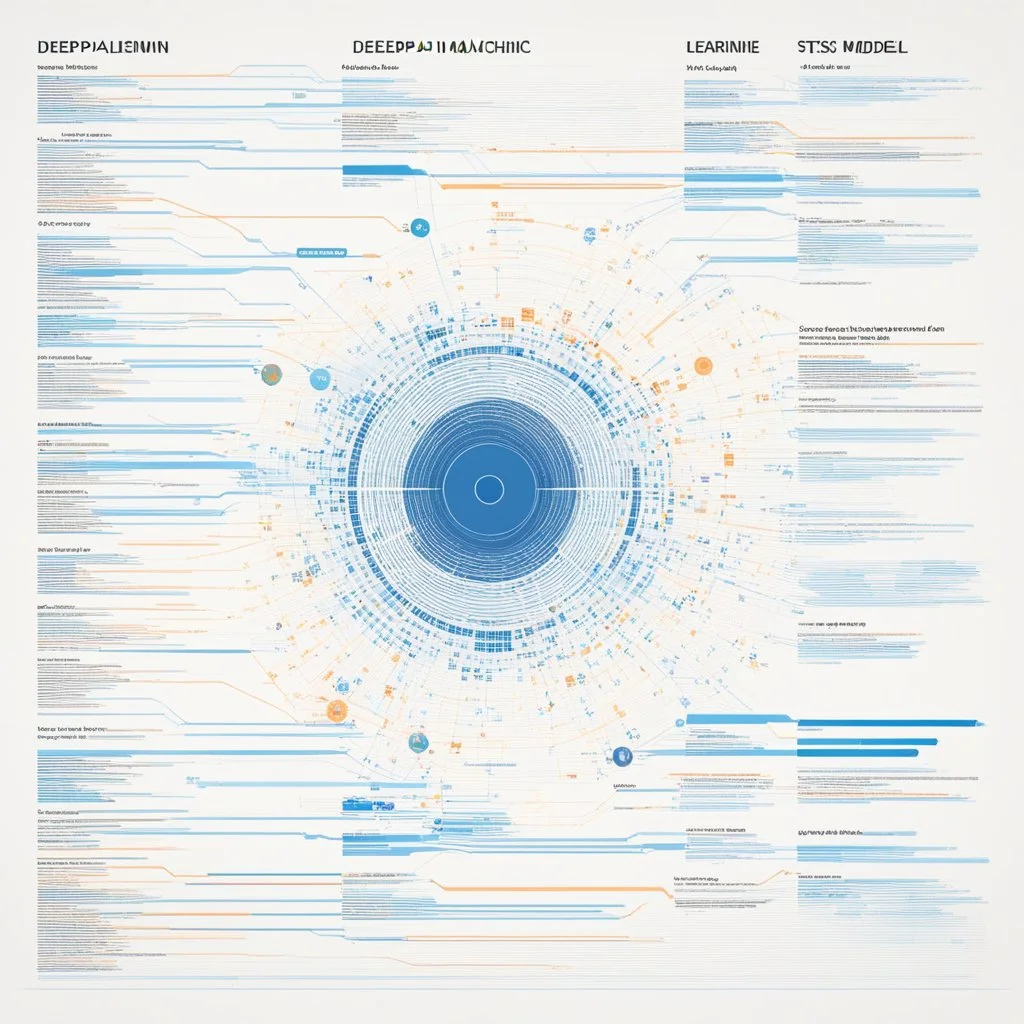
@generalpha
Prompt
https://deepai.org/machine-learning-model/text2img
statue, doubles, twins, entangled fingers, Worst Quality, ugly, ugly face, watermarks, undetailed, unrealistic, double limbs, worst hands, worst body, Disfigured, double, twin, dialog, book, multiple fingers, deformed, deformity, ugliness, poorly drawn face, extra_limb, extra limbs, bad hands, wrong hands, poorly drawn hands, messy drawing, cropped head, bad anatomy, lowres, extra digit, fewer digit, worst quality, low quality, jpeg artifacts, watermark, missing fingers, cropped, poorly drawn
2 months ago
Model
SSD-1B
Guidance Scale
7
Dimensions
1024 × 1024